Domain Generalization: Evolution, Breakthroughs and Future Horizon
Half-day Workshop at CVPR 2025
11th June, 2025
Location: Nashville
MOTIVATION
DG has established itself as a vital and viable research approach for mitigating domain shifts. This
method is particularly appealing because it makes minimal assumptions about target domain data
and eliminates the need for re-training or adaptation steps. As a result, DG is increasingly crucial
for enhancing the robustness of AI models in real-world applications. Despite recent advancements
in visual domain generalization, the scope of research has predominantly been limited to single tasks
(such as classification or segmentation), single modalities (specifically images), straightforward
domain shifts, and closed-world scenarios. However, real-world applications often involve more
complex conditions, including multiple modalities, diverse tasks, advanced neural architectures,
extreme environmental conditions, and resource-limited scenarios—all areas that have seen limited
exploration in DG research. In this workshop, we aim to expand the boundaries of DG by fostering
new ideas and stimulating discussion on these relatively unexplored and challenging aspects of
domain generalization.
- Vision-language foundation models are increasingly used as a backbone in many downstream tasks. These models are designed to generalize across different tasks; however, they get challenged under domain shifts. The workshop will explore how the domain generalization capability of foundational models can be improved in many downstream tasks with significant domain gaps. This is particularly important because these foundational models are finding their way into many real-world applications, such as healthcare, autonomous driving, and climate modeling, where domain shifts are ubiquitous, and model failure could lead to disastrous consequences.
- Multimodal computer vision is becoming popular where multiple modalities, e.g., images, video, text, etc., are used together to learn a model. With various modalities, the challenges of domain generalization are also becoming more pronounced, where the model is required to be robust to domain shifts in each modality it processes. The workshop investigates the complexities of learning a cross-domain generalizable multimodal model across domains and diverse modalities.
- The current progress in domain generalization is mainly limited to images, 2D data, and classification and segmentation tasks. Real-world applications often require processing videos and 3D data and building on tasks beyond classification, such as visual question answering (VQA) and object detection. Furthermore, a somewhat restrictive assumption made in many existing DG methods is closed-set categories and fully labeled training data availability. The workshop will invite new DG methods that can handle newer tasks, modalities, and novel classes and be able to learn with limited labeled training data. Such a new generation of DG algorithms will likely require substantial involvement of cross-disciplinary researchers.
- A vital aspect of workshop is the relevance of considering DG as an essential aspect of AI reliability and safety, particularly when combined with model calibration, fairness, and trustworthiness. Such models will be robust to unseen complex environments and will be able to produce reliable uncertainty estimates. Discussion and research in this direction will interest researchers and industry practitioners beyond vision.
- Finally, the workshop carries significant societal relevance as we intend to encourage AI models that are more inclusive and equitable. For instance, DG algorithms can be combined with bias mitigation techniques to realize models that can perform consistently across various demographic groups and geographic regions, particularly when training data underrepresents some groups or is limited. Such methods can lead to improved transparency in high-stakes decision-making, consequently helping to build accountability and trust, especially in domains where fairness is critical, such as healthcare or public policy.
INVITED SPEAKERS
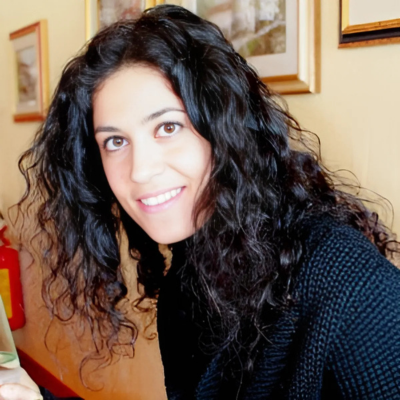
Elisa Ricci
University of Trento
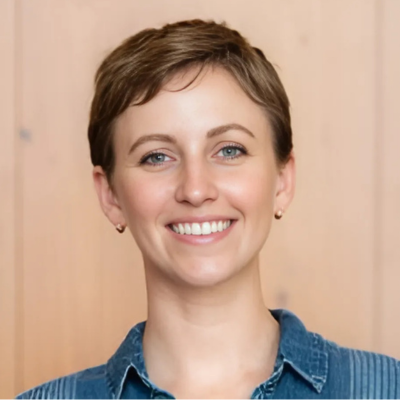
Sara Beery
CSAIL, MIT
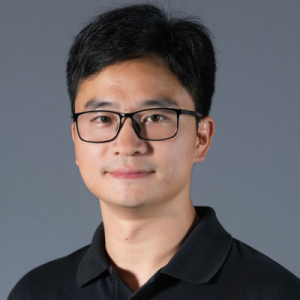
Kai Han
University of Hong Kong
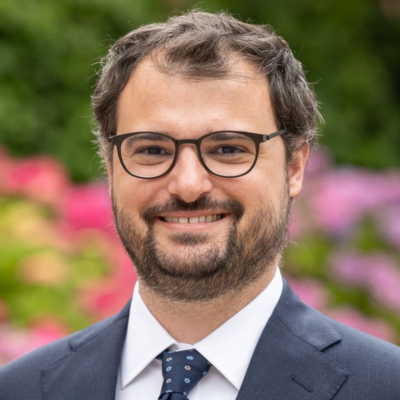
Francesco Locatello
Institute of Science and Technology Austria
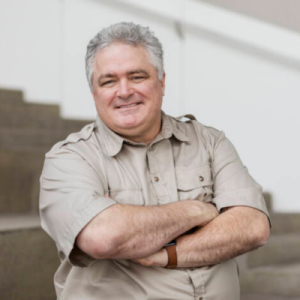
Eric Granger
ETS Montreal Canada
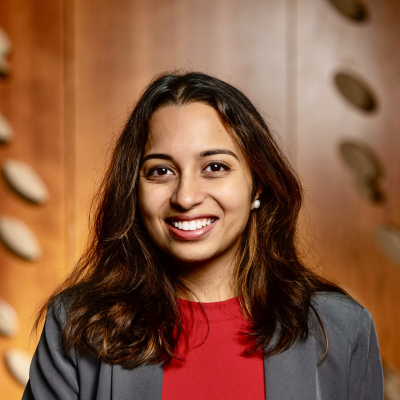
Aditi Raghunathan
Carnegie Mellon University USA
Organizers
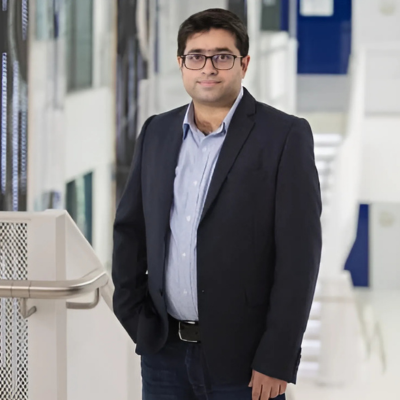
Muhammad Haris Khan
MBZUAI, UAE
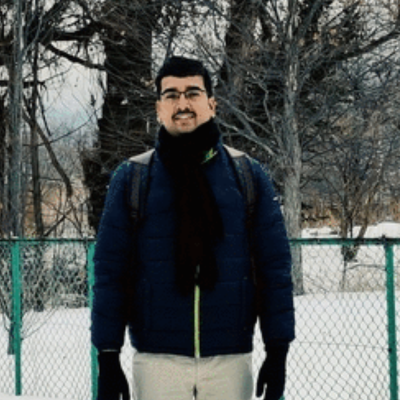
Biplab Banerjee
Indian Institute of Technology Bombay (IIT-B), India
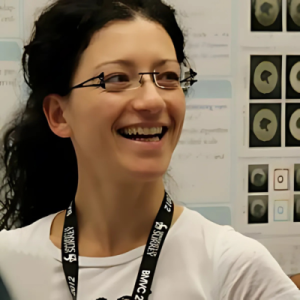
Tatiana Tommasi
Politechnico di Torino Italy, Canada
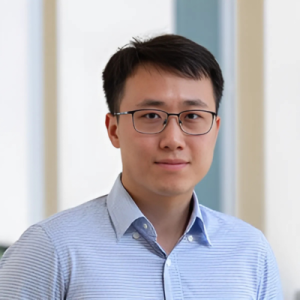
Kaiyang Zhou
HongKong Baptist University HongKong
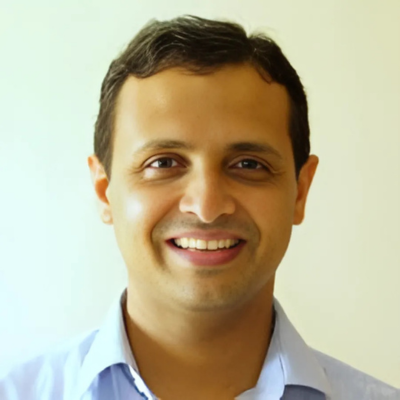
Vineeth Balasubramanian
Indian Institute of Technology Hyderabad (IIT-D), India
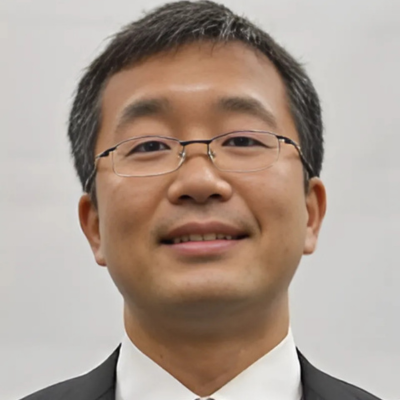
Masashi Sugiyama
RIKEN and the University of Tokyo
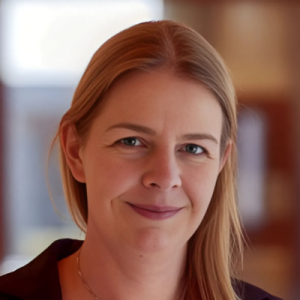
Hilde Kuehne
Tuebingen AI Center Germany
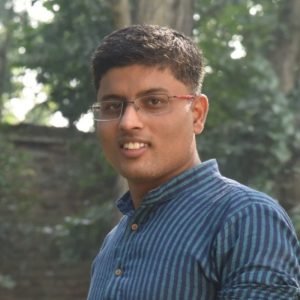
Ankit Jha
LNMIIT Jaipur India
We encourage submissions that are under one of the topics of interest.
- Expanding Domain Generalization Beyond Classification and Images.
- Tackling Extreme Shifts and Long-tail Events.
- Integrating Domain Generalization with Model Calibration.
- Generalizing Multimodal Models Across Domains
- Domain Generalization in Open-World Settings:
- Enhancing Multitask Model Generalization.
- Domain Generalization Beyond Natural Images and Supervised Settings.
Paper Submission:
Please submit your papers at: https://cmt3.research.microsoft.com/DGEBF2025/
Contact:
For questions you can contact us at: muhammad.haris@mbzuai.ac.ae
Sponsors: